Most of my teaching has been in quantitative research methods, including both undergraduate and gradaute courses. I teach courses in the programs in political science and social data analytics at Penn State. Below I provide the syllabi for courses I have been teaching recently.
Course Syllabi
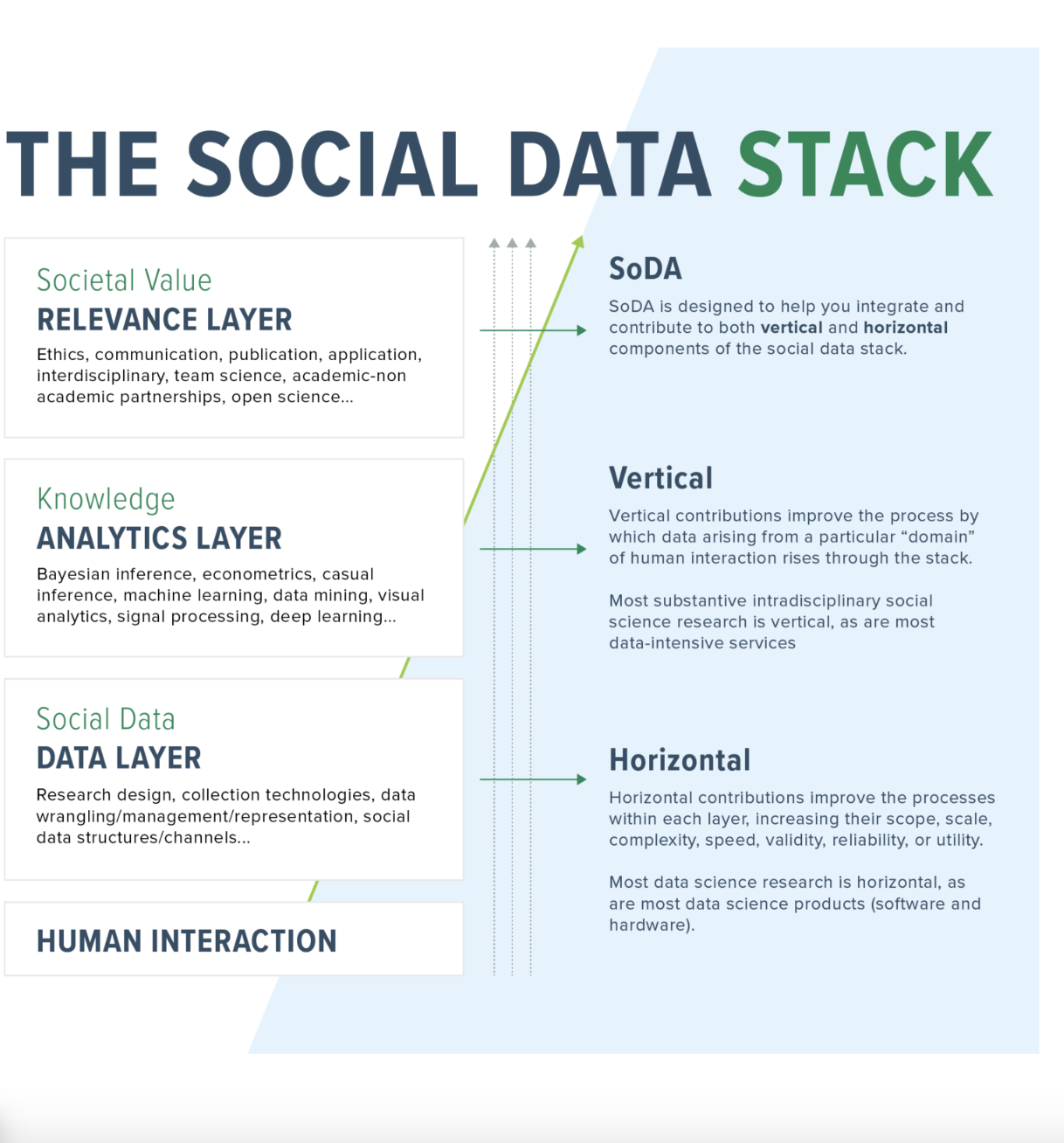
|
SoDA 501: Approaches and Issues in Big Social Data
This seminar is part of the core sequence for students in the Social Data Analytics dual-title PhD and doctoral minor. The primary objective of the seminar is interdisciplinary exposure to, engagement with, and integration of the tools, practices, language, and standards used in the collection and management of data in the component disciplines of the Social Data Analytics field. Each of you is well on your way toward a PhD– formal certification as an “expert”– in one of the component disciplines of Social Data Analytics and has in your coursework and research become well versed in one or more of the many computational, informational, statistical, visual analytic, or social scientific approaches to data, and the issues faced by those approaches. Here, we are interested in trying to integrate your multidisciplinary expertise, particularly in the context of data that are social (about, or arising from, human interaction) and big or intensive (of sufficient scale, variety, or complexity to strain the informational, computational, or cognitive limits of conventional approaches to data collection, management, manipulation, or analysis).
Syllabus
|
|
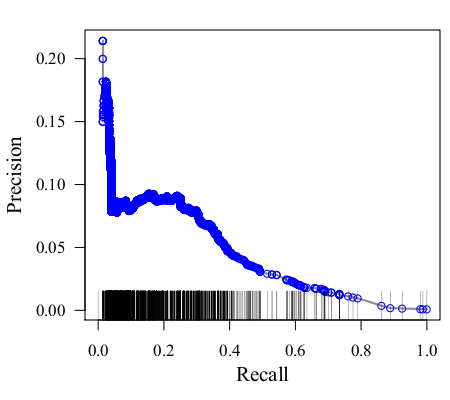
|
Machine Learning for Political Science
Political science research is now regularly conducted using data that is larger and more complex than the data for which conventional statistical tools were designed. Examples of such data include population-scale information on individual-level consumer and political behavior, data streams collected from social media, and archives of electronic government records. There are three fundamental ways in which fine-grained, voluminous, and high-dimensional data require a set of methods that are more flexible than the conventional toolkit of quantitative social science. First, the data is inherently more complex, making it difficult to specify an adequate statistical model from theory alone. Second, the data is high dimensional, meaning there are more variables than one can include in conventional statistical models. Third, the data contains adequate information to make accurate predictions about unseen data (e.g., forecasts). These three features demand a statistical toolkit that is capable of learning model structure, selecting variables, and producing accurate predictions, which are all capabilities of foundational machine learning methods. In this course, we will cover foundational machine learning, with a focus on application to problems in political science.
Syllabus
|
|
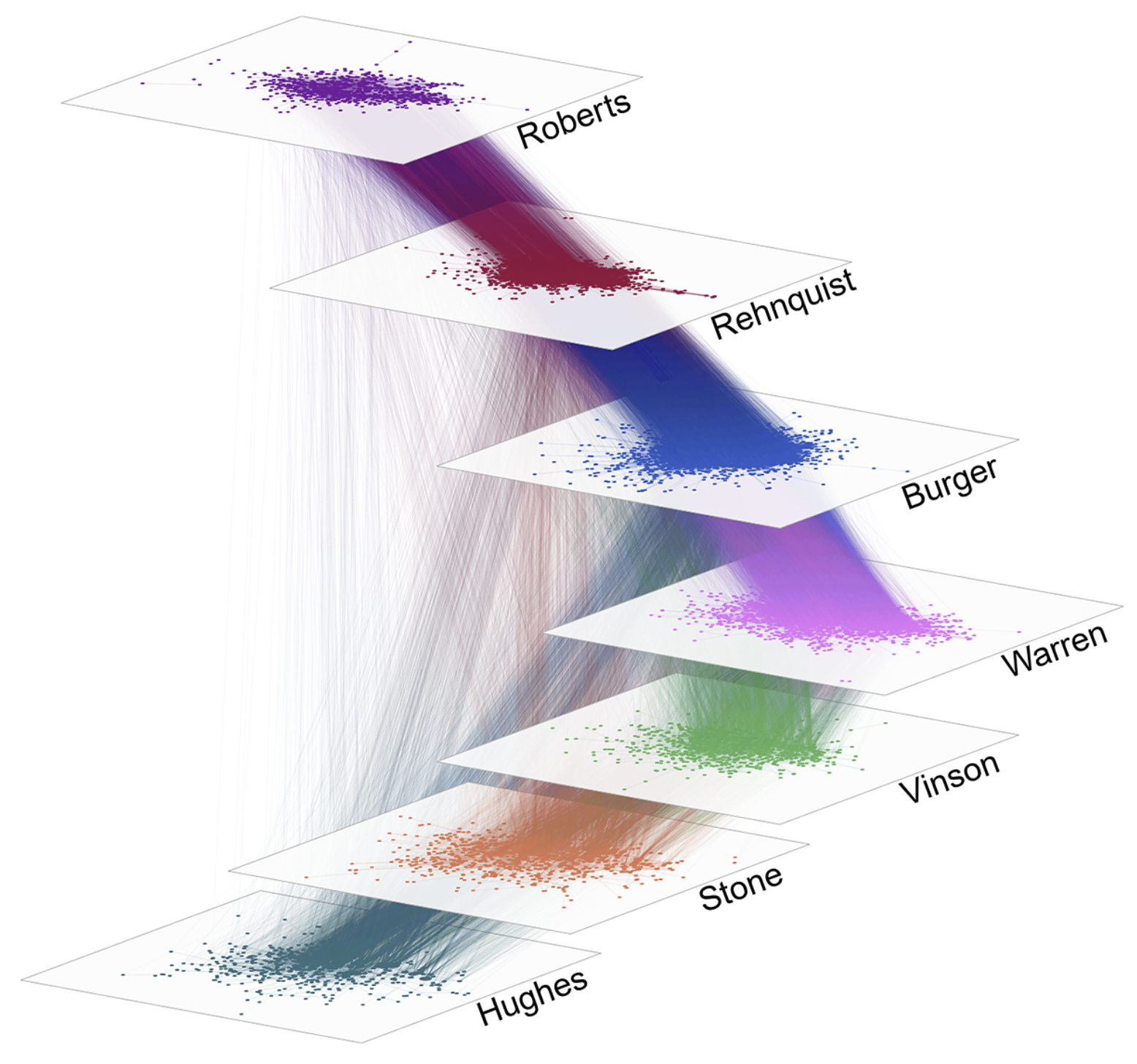
|
Political Networks
A network is a set of relationships among units. The study of networks in political science, the social sciences, and beyond has grown rapidly in recent years. This course is a comprehensive introduction to methods for analyzing network data. We will cover network data collection and management, the formulation and expression of network theory, network visualization and description; and methods for the statistical analysis of networks. The course will make extensive use of real-world applications and students will gain a thorough background in the use of network analytic software. Most of the applications discussed will be drawn from political science and sociology, but this course will be relevant to anyone interested in the study of network data.
Syllabus
|
|
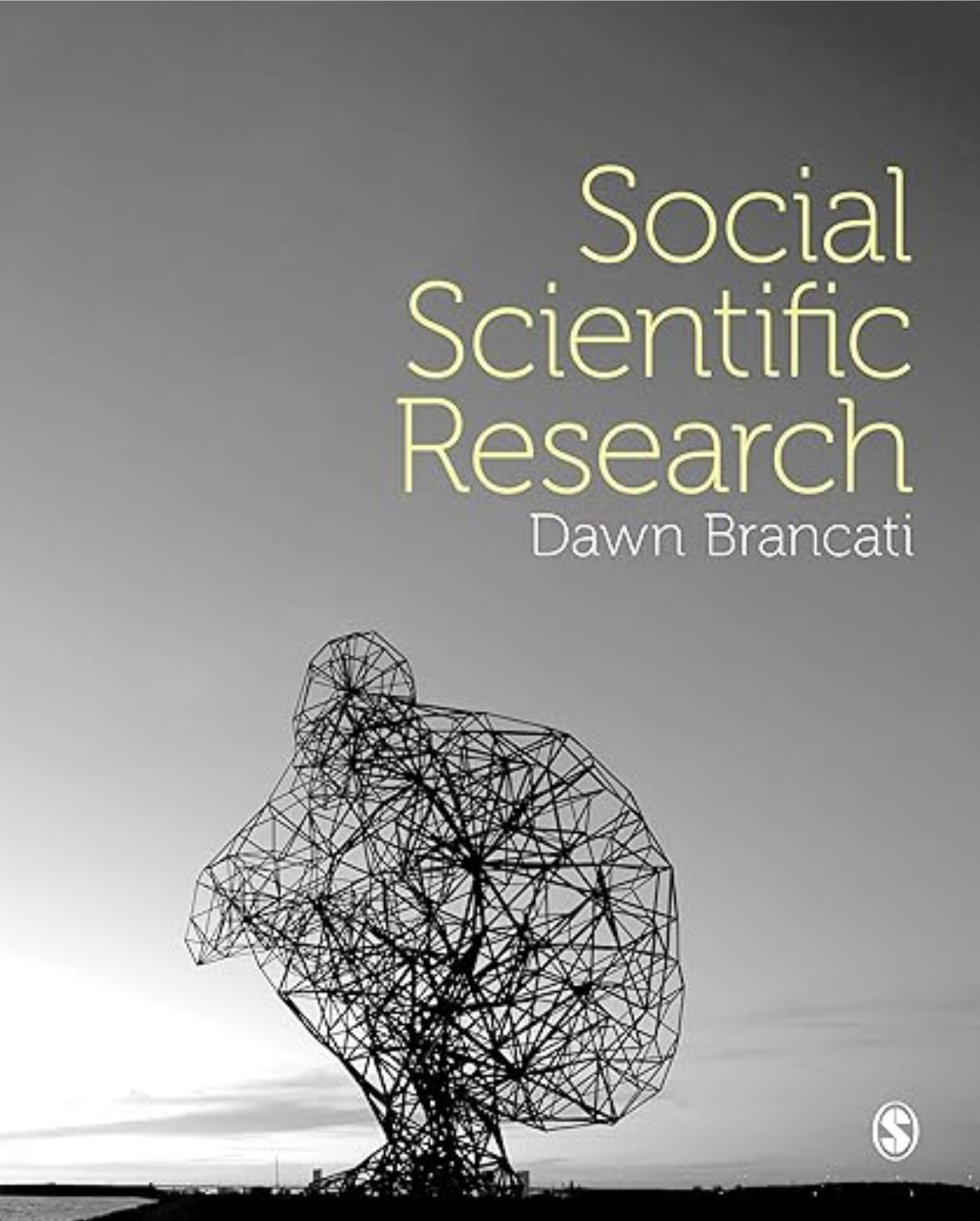
|
SoDA 308: Research Design for Social Data Analytics
This course engages students in the study and use of research design tools for the analysis of social systems and “big data”. Topics to be addressed include: how the scientific method relates to a practice of establishing the validity of propositions and the role that analytics can play in that process when the observations are vast and varied; how the validity of systematic patterns in data are assessed as well as how spurious or biased patterns in the data are ruled out; and how the scientific method can guide the use of exploratory techniques. Through the course, students will learn to develop innovative research designs in an effort to improve the statistical analyses used with social data and how to present these analyses to nontechnical audiences, such as non-profits, employers, and the general public.
Syllabus
|
|
|